Inputs & Discussions
From Theory to Practice
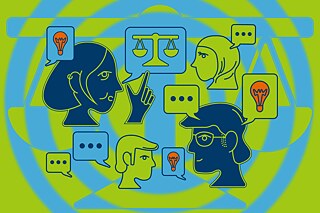
Mon, 26.09.2022 11:30 AM - 12:30 PM
Online
This session is an introduction into Artificial Intelligence and into working with AI.
Schedule
11:30 Christoph Benzmüller (University of Bamberg): What is AI?
The field of artificial intelligence (AI) has already experienced various winter and summer periods in its comparatively young history. The current “heat wave” seems to be stronger and more sustained than previous summer periods, as significant progress has been made in the last two decades, especially in the area of subsymbolic AI.* Data-driven machine learning methods, particularly deep learning, have been hyped in the media, and industry is desperately seeking and hiring experts, since AI is widely considered the steam engine of the 21st century. These success stories have even led to the term AI being largely reduced to subsymbolic AI in the public perception today. At the same time, symbolic AI is now often referred to as good old fashioned AI (GOFAI), which is clearly a misleading term, as both symbolic and subsymbolic AI techniques have been explored as relevant from the beginning, particularly with respect to visions of strong AI. And as we will learn, there are success stories to report in the area of symbolic AI as well, although not at the level of subsymbolic AI, which currently promises to be a very robust and viable choice for many low-hanging fruit applications in industry.
The vision of strong AI, i.e., AI that surpasses human capabilities in all or almost all domains, requires, in my view, the hybridisation of techniques from both sides (or a convincing explanation of why symbolic reasoning techniques should suddenly and readily evolve from data-driven subsymbolic techniques). It is thus in fact the area of hybrid AI where the next “big (intellectual) thing” is to expected. To better understand my viewpoint and to see why I continue to insist on the relevance of symbolic AI, it appears useful that I briefly present my personal working definition of the term AI:
Artificial intelligence is a science of computer technologies developed to achieve and explain intelligent behaviour in machines. By intelligent I refer here to a collection of abilities that enable an entity to (i) solve or learn how to solve certain (difficult) domain specific problems, (ii) master the known and the unknown, i.e., to act successfully in known, unknown, and dynamic environments (which requires perception, planning, agency, etc.), (iii) think rationally, avoid contradictions, and explore abstract theories, (iv) self-reflect, recognise self-contradictions, and reconcile one's reasoning with higher-level goals and norms, and (v) interact socially with other entities and reconcile one's goals and norms with those of a community (for a greater good).
Excellent progress in the field of AI has been made primarily at level (i) and to some extent at level (ii); this progress at both levels has been enabled by both subsymbolic and symbolic techniques, although the emphasis at present, especially at level (i), seems to be more on the former. Progress at the levels (iii)-(v), in my view, clearly requires symbolic modelling and reasoning skills in an intelligent entity; in particular, the exploration and subsequent evaluation of an abstract theory, say in mathematics, traditional natural sciences, metaphysics or law & ethics, inevitably involves mastering a symbolic representation language.
(*: Since its very beginnings, the field of AI has differentiated between the connectionist/subsymbolic and the symbolic paradigms for modelling and explaining intelligent behaviour. Subsymbolic approaches generally model intellectual abilities using artificial neural networks, i.e., networks of computing units devoid of semantic meaning. On the other hand, the symbolic approach assumes that intelligence results from the manipulation of abstract compositional and meaningful representations. Techniques used in this field include rule-based systems and formal logic. Both paradigms have well known strengths and weaknesses, and the debate about whether human-level intelligence can be plausibly modeled and explained by one or the other has a long tradition.)
See also Christoph Benzmüller's forthcoming article: Symbolic AI and Gödel’s Ontological Argument, Zygon, 2022. DOI: 10.1111/zygo.12830
11:45 Q&A/ Discussion
12:00 Alexander Machado (appliedAI): Introduction to the Machine Learning Life Cycle
AI has moved beyond the initial hype and has now reached production in multiple systems across the world. Unfortunately, Gartner estimates that more than 80% of AI projects fail to reach production.
The ML lifecycle is a simplified high-level framework which shows the essential aspects and steps that need to be considered to bring an AI project from conception to production.
This framework addresses typical challenges such as having a research mindset in production setups, poor planning, not having an unified view of the ML lifecycle across teams, inconsistent artifacts management, lack of proper monitoring, automation after deploying a model, and many more.
12:15 Q&A/ Discussion