“Ethics at arm's length”: Kate Crawford book excerpt
In her latest book Atlas of AI leading scholar Kate Crawford writes on how the global networks underpinning artificial intelligence (AI) technology are damaging the environment, entrenching inequality and fuelling a shift toward undemocratic governance.
Whether it’s online translation tools arbitrarily assigning genders to certain professions or facial recognition software misidentifying black faces, there are numerous examples these days of bias generated by artificial intelligence (AI).
Most AI systems reflect characteristics of the dominant voice in their code and in the data they use to learn, and that is male and white. But this process of bias didn’t just show up overnight, it’s the result of decades of developments in the field, as researchers slowly disconnected with the subjects they were investigating according to Australian AI expert Kate Crawford. It’s a process that creatives have recently begun using to inspire their art, as people such as Caroline Sinders and Joy Buolamwini are showing.
In her new book Atlas of AI, Crawford examines the burgeoning growth of AI in an environment where “ethical questions (are separated) away from the technical.” The following excerpt from her book is entitled “Ethics at arm’s length.”
-
The great majority of university-based AI research is done without any ethical review process. But if machine learning techniques are being used to inform decisions in sensitive domains like education and health care, then why are they not subject to greater review? To understand that, we need to look at the precursor disciplines of artificial intelligence. Before the emergence of machine learning and data science, the fields of applied mathematics, statistics, and computer science had not historically been considered forms of research on human subjects.
In the early decades of AI, research using human data was usually seen to be a minimal risk. Even though datasets in machine learning often come from and represent people and their lives, the research that used those datasets was seen more as a form of applied math with few consequences for human subjects. The infrastructures of ethics protections, like university-based institutional review boards (IRBs), had accepted this position for years. This initially made sense; IRBs had been overwhelmingly focused on the methods common to biomedical and psychological experimentation in which interventions carry clear risks to individual subjects. Computer science was seen as far more abstract.
Once AI moved out of the laboratory contexts of the 1980s and 1990s and into real-world situations—such as attempting to predict which criminals will reoffend or who should receive welfare benefits—the potential harms expanded. Further, those harms affect entire communities as well as individuals. But there is still a strong presumption that publicly available data sets pose minimal risks and therefore should be exempt from ethics review. This idea is the product of an earlier era, when it was harder to move data between locations and very expensive to store it for long periods. Those earlier assumptions are out of step with what is currently going on in machine learning. Now datasets are more easily connectable, indefinitely repurposable, continuously updatable, and frequently removed from the context of collection.
Once AI moved out of the laboratory and into real-world situations the potential harms expanded
| Photo: Jürgen Jester / Unsplash
The risk profile of AI is rapidly changing as its tools become more invasive and as researchers are increasingly able to access data without interacting with their subjects. For example, a group of machine learning researchers published a paper in which they claimed to have developed an “automatic system for classifying crimes.” In particular, their focus was on whether a violent crime was gang-related, which they claimed their neural network could predict with only four pieces of information: the weapon, the number of suspects, the neighbourhood, and the location. They did this using a crime dataset from the Los Angeles Police Department, which included thousands of crimes that had been labelled by police as gang-related.
Gang data is notoriously skewed and riddled with errors, yet researchers use this database and others like it as a definitive source for training predictive AI systems. The CalGang database, for example, which is widely used by police in California, has been shown to have major inaccuracies. The state auditor discovered that 23 percent of the hundreds of records it reviewed lacked adequate support for inclusion. The database also contained forty-two infants, twenty-eight of whom were listed for having “admitting to being gang members.” Most of the adults on the list had never been charged, but once they were included in the database, there was no way to have their name removed. Reasons for being included might be as simple as chatting with a neighbour while wearing a red shirt; using these trifling justifications, Black and Latinx people have been disproportionately added to the list.
The CalGang database, which is widely used by police in California, has been shown to have major inaccuracies
| Credit: Sean Lee / Unsplash
When the researchers presented their gang-crime prediction project at a conference, some attendees were troubled. As reported by Science, questions from the audience included, “How could the team be sure the training data were not biased to begin with?” and “What happens when someone is mislabelled as a gang member?” Hau Chan, a computer scientist now at Harvard University who presented the work, responded that he couldn’t know how the new tool would be used. “[These are the] sort of ethical questions that I don’t know how to answer appropriately,” he said, being just “a researcher.” An audience member replied by quoting a lyric from Tom Lehrer’s satiric song about the wartime rocket scientist Wernher von Braun: “Once the rockets are up, who cares where they come down?”
This separation of ethical questions away from the technical reflects a wider problem in the field, where the responsibility for harm is either not recognized or seen as beyond the scope of the research. As Anna Lauren Hoffman writes: “The problem here isn’t only one of biased datasets or unfair algorithms and of unintended consequences. It’s also indicative of a more persistent problem of researchers actively reproducing ideas that damage vulnerable communities and reinforce current injustices. Even if the Harvard team’s proposed system for identifying gang violence is never implemented, hasn’t a kind of damage already been done? Wasn’t their project an act of cultural violence in itself?” Sidelining issues of ethics is harmful in itself, and it perpetuates the false idea that scientific research happens in a vacuum, with no responsibility for the ideas it propagates.
AI scientist Joseph Weizenbaum wrote in 1976 that computer science was already seeking to circumvent all human contexts
| Credit: Chris Barbalis / Unsplash
The reproduction of harmful ideas is particularly dangerous now that AI has moved from being an experimental discipline used only in laboratories to being tested at scale on millions of people. Technical approaches can move rapidly from conference papers to being deployed in production systems, where harmful assumptions can become ingrained and hard to reverse.
Machine learning and data-science methods can create an abstract relationship between researchers and subjects, where work is being done at a distance, removed from the communities and individuals at risk of harm. This arm’s-length relationship of AI researchers to the people whose lives are reflected in datasets is a long-established practice. Back in 1976, when AI scientist Joseph Weizenbaum wrote his scathing critique of the field, he observed that computer science was already seeking to circumvent all human contexts. He argued that data systems allowed scientists during wartime to operate at a psychological distance from the people “who would be maimed and killed by the weapons systems that would result from the ideas they communicated.” The answer, in Weizenbaum’s view, was to directly contend with what data actually represents: “The lesson, therefore, is that the scientist and technologist must, by acts of will and of the imagination, actively strive to reduce such psychological distances, to counter the forces that tend to remove him from the consequences of his actions. He must — it is as simple as this — think of what he is actually doing.”
Kate Crawford
| Photo: Cath Muscat
Weizenbaum hoped that scientists and technologists would think more deeply about the consequences of their work — and of who might be at risk. But this would not become the standard of the AI field. Instead, data is more commonly seen as something to be taken at will, used without restriction, and interpreted without context. There is a rapacious international culture of data harvesting that can be exploitative and invasive and can produce lasting forms of harm. And there are many industries, institutions, and individuals who are strongly incentivized to maintain this colonizing attitude — where data is there for the taking—and they do not want it questioned or regulated.
This excerpt from Atlas of AI is re-printed here with the kind permission of Kate Crawford and Yale University Press. The book can be purchased here.
Most AI systems reflect characteristics of the dominant voice in their code and in the data they use to learn, and that is male and white. But this process of bias didn’t just show up overnight, it’s the result of decades of developments in the field, as researchers slowly disconnected with the subjects they were investigating according to Australian AI expert Kate Crawford. It’s a process that creatives have recently begun using to inspire their art, as people such as Caroline Sinders and Joy Buolamwini are showing.
In her new book Atlas of AI, Crawford examines the burgeoning growth of AI in an environment where “ethical questions (are separated) away from the technical.” The following excerpt from her book is entitled “Ethics at arm’s length.”
-
The great majority of university-based AI research is done without any ethical review process. But if machine learning techniques are being used to inform decisions in sensitive domains like education and health care, then why are they not subject to greater review? To understand that, we need to look at the precursor disciplines of artificial intelligence. Before the emergence of machine learning and data science, the fields of applied mathematics, statistics, and computer science had not historically been considered forms of research on human subjects.
In the early decades of AI, research using human data was usually seen to be a minimal risk. Even though datasets in machine learning often come from and represent people and their lives, the research that used those datasets was seen more as a form of applied math with few consequences for human subjects. The infrastructures of ethics protections, like university-based institutional review boards (IRBs), had accepted this position for years. This initially made sense; IRBs had been overwhelmingly focused on the methods common to biomedical and psychological experimentation in which interventions carry clear risks to individual subjects. Computer science was seen as far more abstract.
Once AI moved out of the laboratory contexts of the 1980s and 1990s and into real-world situations—such as attempting to predict which criminals will reoffend or who should receive welfare benefits—the potential harms expanded. Further, those harms affect entire communities as well as individuals. But there is still a strong presumption that publicly available data sets pose minimal risks and therefore should be exempt from ethics review. This idea is the product of an earlier era, when it was harder to move data between locations and very expensive to store it for long periods. Those earlier assumptions are out of step with what is currently going on in machine learning. Now datasets are more easily connectable, indefinitely repurposable, continuously updatable, and frequently removed from the context of collection.
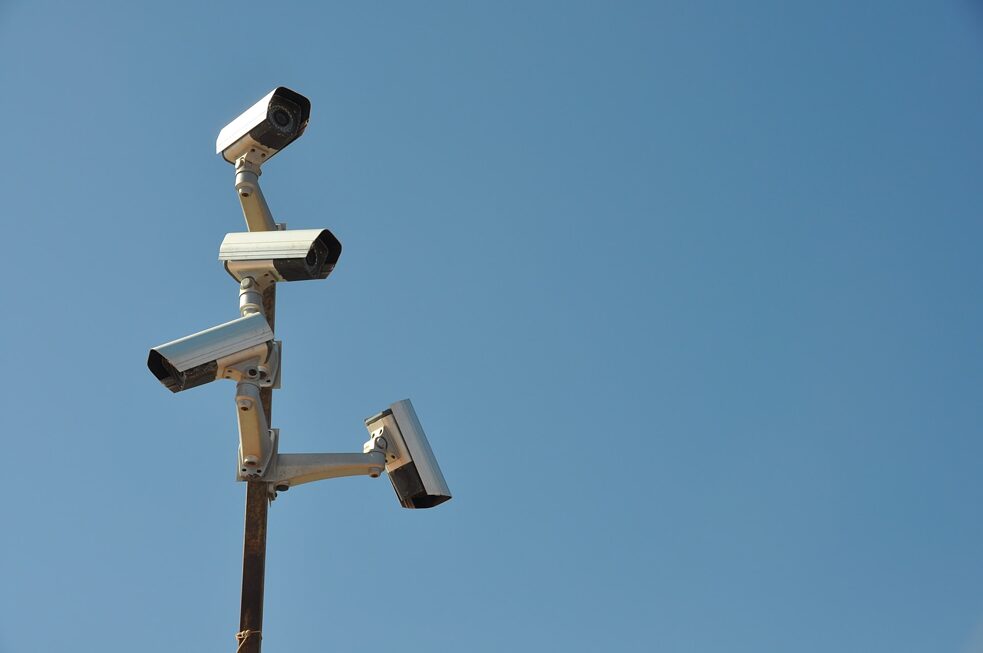
Gang data is notoriously skewed and riddled with errors, yet researchers use this database and others like it as a definitive source for training predictive AI systems. The CalGang database, for example, which is widely used by police in California, has been shown to have major inaccuracies. The state auditor discovered that 23 percent of the hundreds of records it reviewed lacked adequate support for inclusion. The database also contained forty-two infants, twenty-eight of whom were listed for having “admitting to being gang members.” Most of the adults on the list had never been charged, but once they were included in the database, there was no way to have their name removed. Reasons for being included might be as simple as chatting with a neighbour while wearing a red shirt; using these trifling justifications, Black and Latinx people have been disproportionately added to the list.
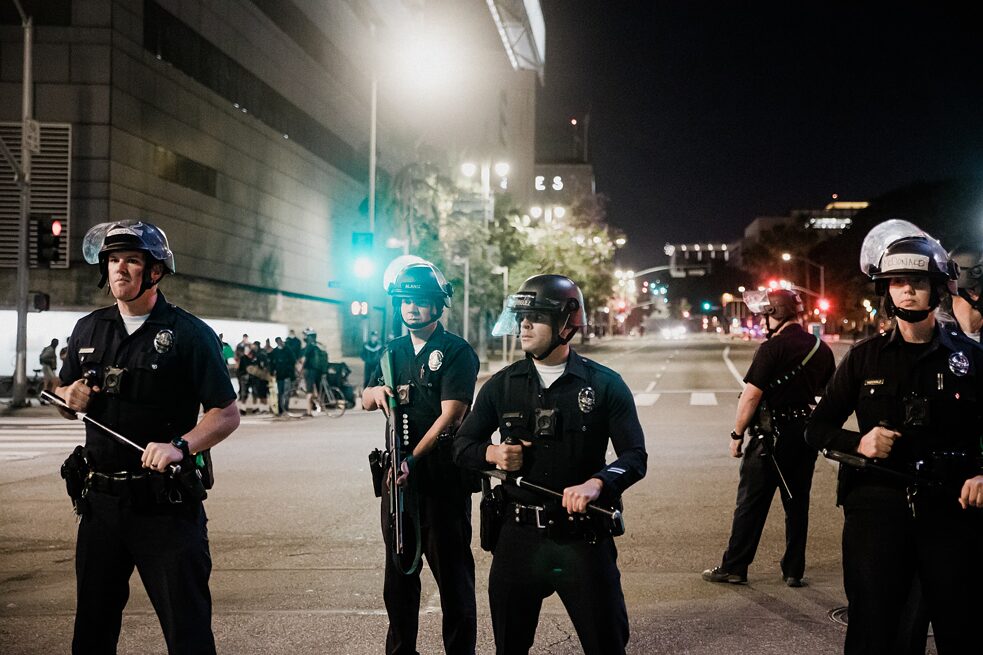
This separation of ethical questions away from the technical reflects a wider problem in the field, where the responsibility for harm is either not recognized or seen as beyond the scope of the research. As Anna Lauren Hoffman writes: “The problem here isn’t only one of biased datasets or unfair algorithms and of unintended consequences. It’s also indicative of a more persistent problem of researchers actively reproducing ideas that damage vulnerable communities and reinforce current injustices. Even if the Harvard team’s proposed system for identifying gang violence is never implemented, hasn’t a kind of damage already been done? Wasn’t their project an act of cultural violence in itself?” Sidelining issues of ethics is harmful in itself, and it perpetuates the false idea that scientific research happens in a vacuum, with no responsibility for the ideas it propagates.
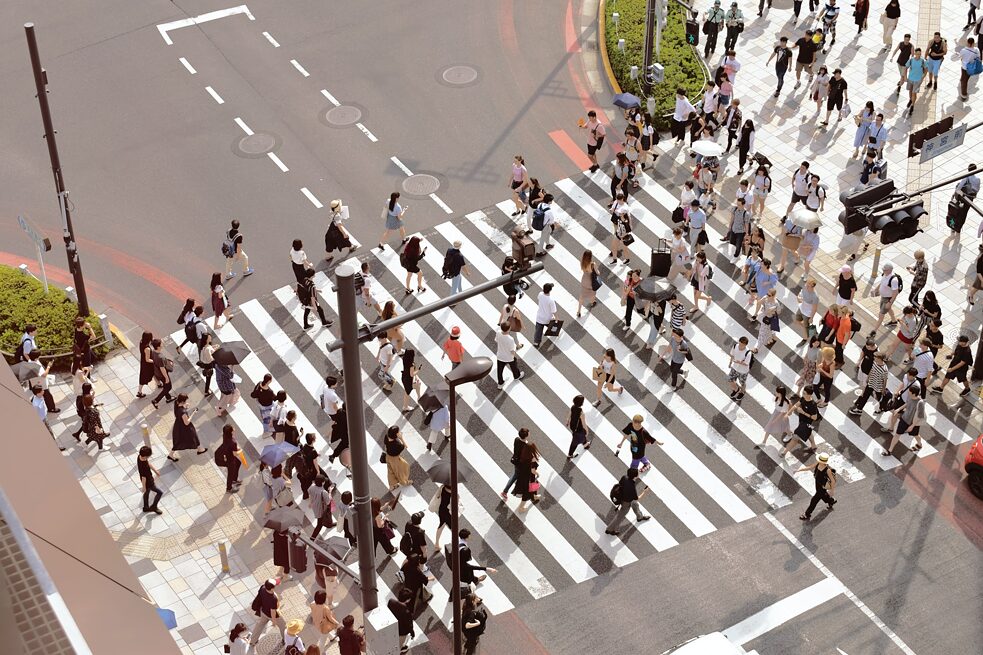
Machine learning and data-science methods can create an abstract relationship between researchers and subjects, where work is being done at a distance, removed from the communities and individuals at risk of harm. This arm’s-length relationship of AI researchers to the people whose lives are reflected in datasets is a long-established practice. Back in 1976, when AI scientist Joseph Weizenbaum wrote his scathing critique of the field, he observed that computer science was already seeking to circumvent all human contexts. He argued that data systems allowed scientists during wartime to operate at a psychological distance from the people “who would be maimed and killed by the weapons systems that would result from the ideas they communicated.” The answer, in Weizenbaum’s view, was to directly contend with what data actually represents: “The lesson, therefore, is that the scientist and technologist must, by acts of will and of the imagination, actively strive to reduce such psychological distances, to counter the forces that tend to remove him from the consequences of his actions. He must — it is as simple as this — think of what he is actually doing.”
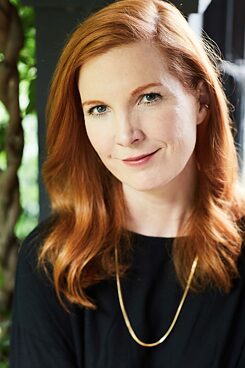
This excerpt from Atlas of AI is re-printed here with the kind permission of Kate Crawford and Yale University Press. The book can be purchased here.