Expert statements
‘The collaboration between humans and machines needs to be redefined.’
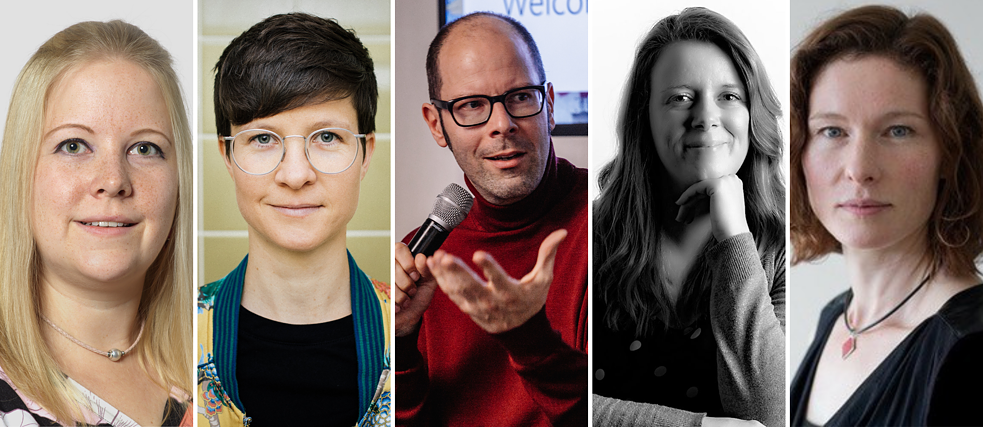
Whenever it comes to fairness or ethically relevant decisions, things become difficult for Artificial Intelligence. ‘An algorithm has no sense of tact’ is how Prof. Dr. Katharina Zweig aptly puts it with the title of her current SPIEGEL bestseller (‘Ein Algorithmus hat kein Taktgefühl’; Heyne Verlag, 2019). In the production of written information, AI holds unfathomable opportunities. Without human reflection, however, they also carry the risk of reproducing stereotypes and – as far as the choice of terminology is concerned, for example in relation to gender and ethnicity – of having a discriminatory effect. Ultimately, Deep Learning and AI are very much like raising a child: you have to teach it what it doesn't know. In that respect, the data with which AI is trained is itself tainted with prejudices. What kinds of bias can be found in texts that were created with the help of AI? And what solutions can be implemented to mitigate or even avoid distortions of reality? We talked about this with five experts from the UK, Germany, the Netherlands, and Switzerland.
By Stephanie Hesse
Prof. Dr. Mascha Kurpicz-Briki
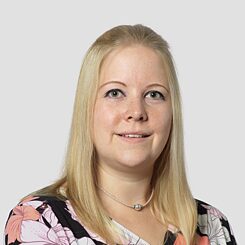
When AI makes decisions about people, and contains or even reinforces society's stereotypes, there can be strong and systematic discrimination when such systems are used.
The solution is very challenging, on the one hand due to the difficult definition of “Fairness”, and on the other hand due to the technical implementation, which is still in the research stage. Therefore, it is important to be aware of these issues and to ask the right questions – both when selecting training data and when deploying the software. The collaboration between humans and machines needs to be redefined, and AI should be a decision-making aid rather than replace humans. In this context, we also talk about “Augmented Intelligence” instead of “Artificial Intelligence”.
Uli Köppen
Uli Köppen: Head of AI + Automation Lab | Co-Head of BR Data. She focuses on the use of Artificial Intelligence in data journalism.
| © Lisa Hinder, BR
Here, algorithms have the potential to strengthen existing biases (prejudices, false weightings) through so-called scaling effects. AI language models can reinforce bias for example, especially if the training texts used already contain such imbalances. If for example gender-neutral language was rarely used, this effect can be amplified by algorithms during automatic text production. Some examples of bias can also be found in automatic translation, when gender stereotypes are reproduced there, such as the translation of “nurse” as “Krankenschwester” (which is a woman in German).
It is therefore important that every industry that uses algorithms is aware of the problems that this technology brings with it. To this end, Bayerischer Rundfunk has created its own AI guidelines that we adhere to. Of course, this does not protect us from errors and blind spots, but it does increase our awareness of any problems.
We at AI + Automation Lab, at BR Data and atBR Recherche look at both sides in the deployment of this technology: with investigative reporting on algorithms, we try to enrich the debate on where and how we as a society want to deploy AI, and also look critically at this technology.
At the same time, we use AI and automation to support our colleagues in their work and to offer our users the best possible reporting. The use of algorithms also offers the chance to hold up a mirror to journalism itself and to check for possible discrimination. An example is the London School of Economics' Aijo Project in which media from all over the world examined their own web presences for diversity and, with the aid of algorithms, determined that women and people of colour are under-represented in their reporting.
Automation is a method – where and how it is used determines whether it helps to discover prejudices or actually reinforces them under certain circumstances.
Dr. Phil. Aljosha Burchardt
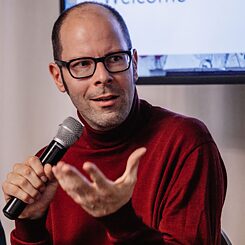
The systems act, if you like, purely syntactically. They have no access to the world other than through the data. Above all, they lack the possibility of (corrective) meta-reflection; a weak AI system cannot ask itself: ‘What am I actually doing here right now?’.
Either, one can largely avoid bias by using suitable data (for example synthetic data), or one makes use of the “human-in-the-loop” principle, i.e. human interaction in the course of data preparation. At some point in the future, we may have hybrid AI systems where one has meaningful access to their “knowledge”.
The use of AI can help us make the world more inclusive: it can translate, not only between different languages, but also into simple language or sign language for instance. AI can search for and prepare information for specific target groups. This offers many opportunities to bring people into the (digital) discourse who are currently excluded.
Dr. Stefanie Ullmann
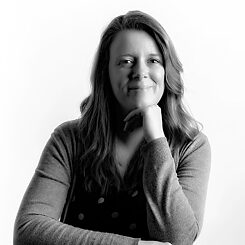
Biases are inherently human. But when they incorrectly represent actual distributions in society and are left unchallenged, they can have a serious negative social impact. Especially when they are reinforced and even amplified by AI. If an automated system is trained on imbalanced data, it will inevitably lead to unfair distribution, systematically disadvantaging groups of people. This can have catastrophic consequences for individuals as, for instance, automated decision-making tools are increasingly used in finance, employment, or health care.
First and foremost, there need to be stricter guidelines for the selection and annotation of training data and we need experts and developers to work together interdisciplinarily at all stages of the development of AI systems. Moreover, we need a more diverse representation of individuals especially amongst annotators. There are also possible solutions for already existing problems such as hate speech online. My colleagues and I, for instance, developed an app that automatically puts suspicious messages and posts into a kind of quarantine, similar to computer virus detection systems. The user then gets a warning as well as an indication of how likely it is that the message contains harmful content. In the end, the user can decide if they wish to view the post or not. Such applications can be used independently of the social media platform.
Laura Hollink
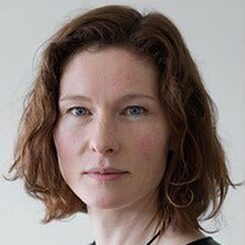
Contentious words in cultural heritage collections can be problematic in two ways. Firstly, they may be offensive when encountered by visitors. Discriminative word usage undermines the role of the heritage institute as a trusted and inclusive source of information. Secondly, heritage collections may be used as input data to train a wide range of AI applications, such as automatic tagging or query auto-completion systems. When training a language model, contentious terms in the training data may result in contentious words in the output.
Cultural heritage institutes have dealt with contentious terms in their collections in various ways depending on their requirements and collection. Some decided to leave them as they are, to ensure an authentic representation of historic viewpoints - others have added explanations of the meaning of these words - in some cases, words have been replaced. AI has the potential to support this process by predicting which terms are potentially contentious on a large scale. This is a challenging task since contentiousness is subjective and dependent on context. When heritage collections are used to train AI systems, it is important to be aware of the (historic) viewpoints ingrained in the data and to be explicit about this towards users of the AI system.
The Culturally Aware AI project has taken a first step in this direction by creating a corpus of contentious terms in context, called “ConConCor.” It consists of 2,715 unique text snippets from historical Dutch newspapers, annotated with information on whether a particular target term in a context is contentious or not. Each text snippet is annotated by at least seven annotators, both experts and crowd annotators, to allow for an in-depth analysis of the inter-rater agreement. We find that while the overall agreement is low, there is a large number of text snippets of which annotators agree. We have used ConConCor as a training set to predict the contentiousness of words. First experiments showed promising results, confirming that both the terms themselves and the context play a role in whether a term is contentious. We see the detection of contentious terms as a first step towards making (historic) perspectives in heritage collections explicit.