Summary of the results
Artificially Correct Hackathon 2021
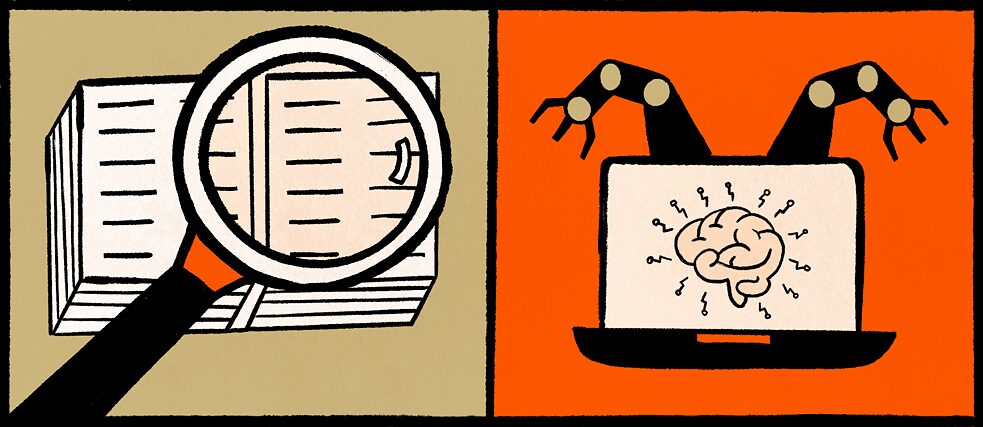
Between the 1st and 3rd of October, translators, activists and software developers from all over the world, came together to participate in the Goethe-Institut’s Artificially Correct Hackathon.12 teams had 52 hours (lots of coffee), and one mission – to develop innovative, forward-looking ideas and projects to help tackle bias in language and machine translators.
By Alana Cullen and Priyanka Dasgupta
The need to hack
Each word has a history, a meaning, a context, and as speakers of language, we have the choice to use these words. Therefore, language is not just words spoken, but a vehicle of social reality. Why say ‘chairman’, as opposed to the more neutral ‘chair’ or ‘chairperson’? Or why, when thinking of a ‘nurse’, automatically presume the pronoun ‘she’? Stereotypes are embedded not only in mindsets but also words.
Consequently, stereotypes also get embedded in artificial intelligence (AI). For instance when translating from Polish to English, Google translate automatically assumes the pronouns for certain tasks when the subject is ambiguous:
“She is beautiful”
“He is clever”
“He reads”
“She washes the dishes”
We see this bias in AI for machine translations because it is trained off data sets that contain human bias, and it learns the default patterns in our language.
Not only does AI have built-in gender bias, but racial too. For example, the term “half blood”, which historically has been used as a racial slur, is now propagated and reinforced based off the popularity of children’s fiction. Consequently, the language bias is perpetuated in technology as AI learns from its maker - and the more prejudice it sees, the more biased the system’s default behaviour will become.
Acknowledging this bias gets us one step closer to making more neutral, unbiased machine translation. Of course, true neutrality is not achievable in a world with so many power imbalances, but certainly we can work towards making AI work better for more people. Working towards solutions to this bias, will help us tap into the potential that digital transformation can provide, for everybody.
Generating solutions
It was these innovative solutions that the participants of the Hackathon focused on. Five challenges or areas of bias in AI were identified, that the teams could ‘hack’ into. These included developing gender-fair post-editing techniques, as well as finding a way to identify sentences that were susceptible to bias in machine translation.
Alongside hacking, participants were also given the opportunity to attend keynote lectures by those working in the field of gender, race and AI studies – Ylva Habel, Dagmar Gromann and Manuel Lardelli, Danielle Saunders and Sheila Beladinejad, who shared their ideas and experience:
A complication for translation bias is language change. Our ideas of gender and gendered language and stereotypes change over time.
Danielle Saunders, Hackathon keynote speaker
The hackers were also provided with access to experts in the AI field, who could support them in generating their solutions.
I had a fun time attending the Hackathon and putting my expertise for creative solutions to tackle bias in AI translations. It was a brilliant way to collaborate with people from different fields, all combining their ideas.
Dr Antje Bothin (she, her), Hackathon participant
All 12 teams did a fantastic job of designing innovative solutions to bias in artificial intelligence. On the final day of the Hackathon, the jury chose five teams to present their ideas in more detail.
The Hackathon brought to front many interesting solutions sprung from the intensive hack sessions. Teams came up with algorithms to measure the ‘distance’ of bias in words susceptible to misgendering or bias; one team presented an idea of an easy gender toggle for post-translation editing. Some focused on the importance of creating more inclusive datasets for AI to train on, while others developed a sort of ‘bias-library’ for this purpose. Another team also developed a tool to allow the user to not only identify gender but also racial susceptibility of words to bias.
From these pitches, two winning teams were chosen to continue generating their solutions beyond the Hackathon.
Looking forward
The issue of bias in AI is bigger than our teams could solve over a weekend, but, we have to start somewhere.
The fantastic range of solutions generated in such a short period of time shows just how possible it is to make the changes you want to see, given the right tools and time. In making AI more inclusive, all our teams helped bring us one step closer to helping AI embody everyone’s voice.
As for the winners of the Hackathon, they will continue to go on developing their solutions further, thanks to their €2500 cash prize, and support through mentoring and the resources of Goethe Institut.
We have still far to go, but each step counts: solutions from the hackathon, continued discussions and collaborative efforts, will help affect change in the way we and AI use our words; words that speak for everyone.
Check out the 5 teams and their solutions to bias in AI shortlisted in our Artificially Correct Hackathon:
Check out the video interviews with the winner teams discussing their projects and learnings from the hackathon: